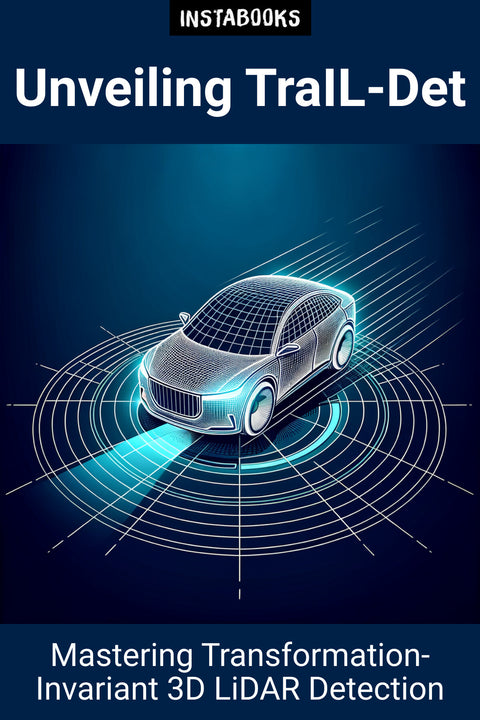
Unveiling TraIL-Det
Mastering Transformation-Invariant 3D LiDAR Detection
Premium AI Book - 200+ pages



Introduction to TraIL-Det
Discover the cutting-edge world of TraIL-Det in this comprehensive guide to transformation-invariant local feature networks for 3D LiDAR object detection. Whether you're a beginner or an expert in the field, this book offers a deep dive into methodologies and applications that are reshaping the landscape of autonomous driving and robotics.Key Innovations in TraIL-Det
Explore how TraIL-Det utilizes unsupervised pre-training to extract meaningful features from raw point cloud data. With a focus on localization, discover how transformation-invariant local features are harnessed through advanced neural network architectures to create robust 3D object detection systems. This chapter unveils the revolutionary approaches that allow the system to maintain consistency across various transformations including translation, rotation, and scaling.Application in Autonomous Driving
Dive into the real-world applications of TraIL-Det in autonomous vehicles. Understand the challenges it addresses such as varied object positioning and scaling, and how its methodology enhances adaptation to the dynamic nature of driving environments. Learn how its architecture improves detection predictability and reliability in diverse scenarios.Recent Developments and Performance Evaluation
Get acquainted with the recent advancements of TraIL-Det tested against benchmark datasets like KITTI and Waymo. This section discusses its superior performance over other self-supervised approaches across different label ratios, underlining its flexibility and effectiveness in diverse operational conditions.Enhancing Robustness Against Rigid Transformations
Understand how transformation-invariance elevates 3D object detection systems, offering improved generalization capabilities to detect various objects in new environments. This chapter breaks down the complex techniques into understandable concepts, making it accessible to a broad audience interested in the future of autonomous systems.See how this powerful technology is shaping the field of computer vision and establishing new standards in 3D perception systems. Each chapter is meticulously researched, presenting unique perspectives and applications that are set to revolutionize autonomous driving.
Table of Contents
1. Introduction to TraIL-Det- The Rise of 3D LiDAR
- Understanding Local Feature Networks
- The Significance of TraIL-Det
2. Foundations of Unsupervised Pre-training
- Learning from Raw Data
- Techniques of Pre-training
- Challenges and Solutions
3. Building Transformation-Invariant Features
- Achieving Invariance
- Neural Networks Architecture
- Implementation Strategies
4. Integrating with Detection Architecture
- The Role of Transformers
- Feature Aggregation Techniques
- Optimizing Predictions
5. Applications in Autonomous Driving
- Overcoming Environmental Challenges
- Real-time Object Detection
- Adapting to Dynamic Scenarios
6. Performance on Benchmark Datasets
- KITTI Dataset Insights
- Waymo Challenges
- Comparative Analyses
7. Recent Advances in TraIL-Det
- Latest Research Contributions
- Technological Innovations
- Future Directions
8. Real-World Implementations
- Industry Case Studies
- Collaborations with Auto Manufacturers
- Robotics Integration
9. Enhancing Robustness and Accuracy
- Addressing Transformation Challenges
- Improving Generalization
- Precision in Detection
10. Potential for Future Development
- Expanding Application Areas
- Innovative Use-Cases
- Pathways to Upgradation
11. Ethical and Societal Implications
- Impact on Autonomous Technology
- Societal Acceptance and Challenges
- Shaping the Future of Mobility
12. Conclusion and Insightful Reflections
- Summary of Key Learnings
- Reflective Thoughts
- Vision for Future Research
Target Audience
This book is written for researchers, developers, and professionals in the fields of computer vision, autonomous driving, and robotics, as well as students interested in advancements in 3D perception technology.
Key Takeaways
- Understanding the innovative methodologies behind TraIL-Det and its impact on 3D LiDAR object detection.
- Insights into unsupervised pre-training and its application in extracting meaningful features from raw data.
- Exploration of transformation-invariant local feature networks and their role in enhancing detection robustness.
- Knowledge of real-world applications in autonomous driving and robotic systems.
- Awareness of recent advancements and performance achievements against benchmark datasets.
- Understanding ethical implications and future potential of TraIL-Det technology.
How This Book Was Generated
This book is the result of our advanced AI text generator, meticulously crafted to deliver not just information but meaningful insights. By leveraging our AI story generator, cutting-edge models, and real-time research, we ensure each page reflects the most current and reliable knowledge. Our AI processes vast data with unmatched precision, producing over 200 pages of coherent, authoritative content. This isn’t just a collection of facts—it’s a thoughtfully crafted narrative, shaped by our technology, that engages the mind and resonates with the reader, offering a deep, trustworthy exploration of the subject.
Satisfaction Guaranteed: Try It Risk-Free
We invite you to try it out for yourself, backed by our no-questions-asked money-back guarantee. If you're not completely satisfied, we'll refund your purchase—no strings attached.